The Tech Behind Self-Driving Cars: A Deep Dive
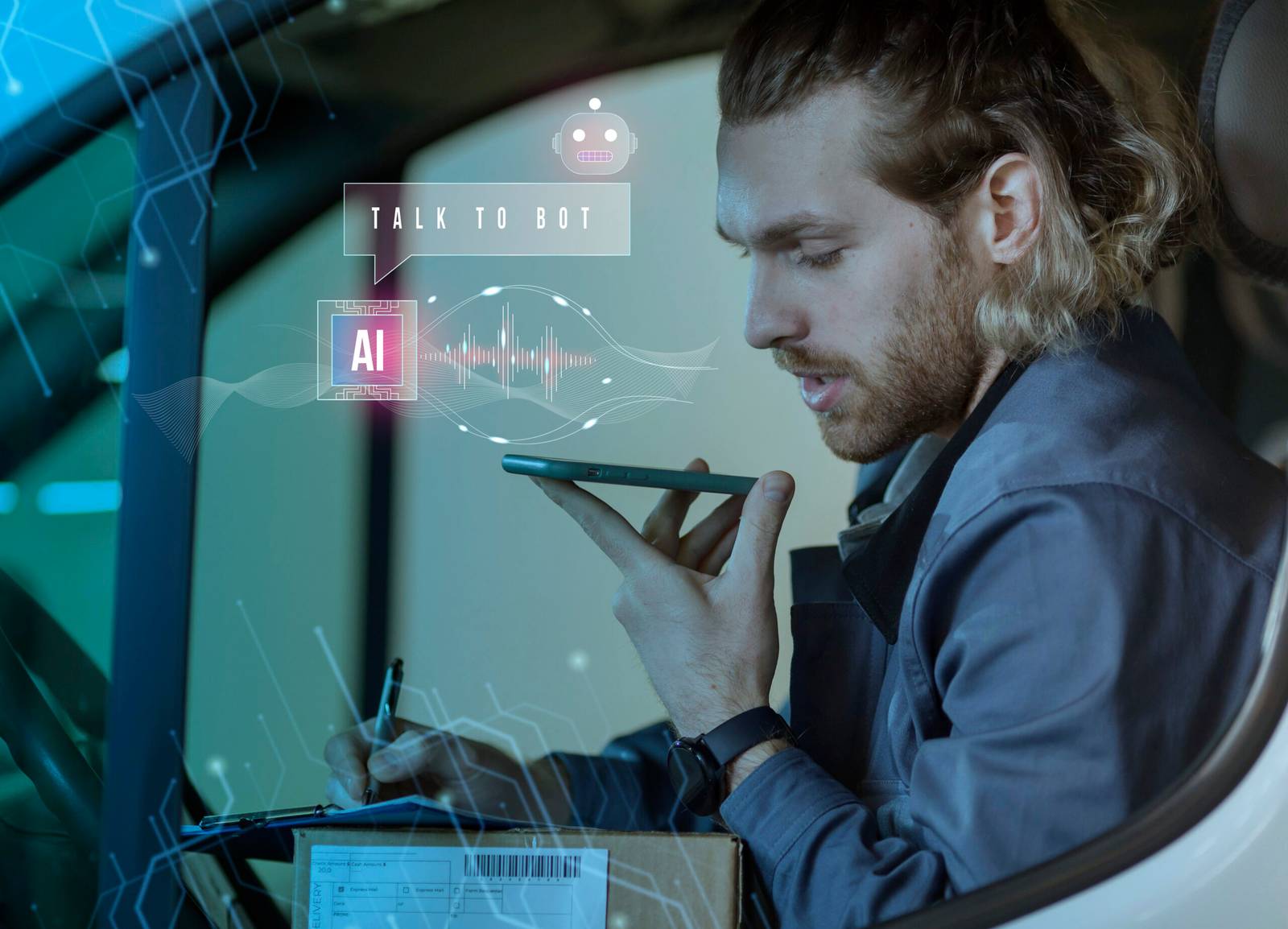

by Web Digital
Self-driving cars, also known as autonomous vehicles, have become a hot topic in recent years. Captivating the imagination of the public and transforming the automotive industry. These vehicles have the potential to revolutionize transportation, making it safer, more efficient, and more convenient. But what’s the technology that makes The Tech Behind Self-Driving Cars: A Deep Dive possible? In this deep dive, we’ll explore the cutting-edge technology behind self-driving cars, The sensors that perceive the environment to the algorithms that control their movements.
Sensors: Eyes and Ears of Self-Driving Cars
At the heart of every self-driving car is an array of sensors that serve as the vehicle’s eyes and ears. These sensors provide real-time data about the vehicle’s surroundings, allowing it to navigate, make decisions, and respond to changing conditions. The most common types of sensors used in autonomous vehicles include:
LiDAR (Light Detection and Ranging): LiDAR sensors use laser beams to measure the distance between the car and objects in its vicinity. By emitting thousands of laser pulses per second. LiDAR systems create a detailed 3D map of the car’s environment, enabling it to identify other vehicles, pedestrians, and obstacles.
Cameras: High-resolution cameras capture images and videos of the vehicle’s surroundings. These images are processed by computer vision algorithms to detect and identify objects, road signs, traffic lights, and lane markings.
Radar: Radar sensors use radio waves to measure the distance and speed of objects in the car’s path. They are particularly useful in adverse weather conditions, such as heavy rain or fog. Where other sensors like LiDAR or cameras might struggle.
Ultrasonic sensors: These sensors use sound waves to detect objects in close proximity to the vehicle. Which is helpful for parking and low-speed maneuvers.
GPS and IMU: Global Positioning System (GPS) and Inertial Measurement Unit (IMU) sensors help the car determine its precise location and orientation on the road.
Data Fusion: Making Sense of the Sensor Data
Self-driving cars generate an enormous amount of data from their sensors, and making sense of this data is a complex task. Data fusion, a critical technology in autonomous vehicles. Integrates information from multiple sensors to create a comprehensive and accurate representation of the vehicle’s surroundings. This fused data is then used for decision-making and navigation.
The data fusion process involves aligning and synchronizing data from different sensors and removing noise or inaccuracies. Advanced software algorithms analyze the combined data to create a precise understanding of the environment. Such as the position and speed of other vehicles, the location of pedestrians, and the condition of the road.
Mapping and Localization
Mapping and localization are fundamental for self-driving cars to know where they are and where they need to go. High-definition maps, combined with real-time sensor data, help the vehicle establish its position on the road and determine the best path to reach its destination.
Maps used in autonomous driving are far more detailed than traditional maps, containing information about lane boundaries, traffic signs, traffic lights, and even the curvature of the road. These maps are continually updated to reflect changes in the environment.
For localization, the car’s sensors compare the real-time data they capture with the data from the map to determine the vehicle’s exact location. Advanced algorithms are used to correct any discrepancies and provide precise positioning.
Perception and Object Detection
Perception is the process through which a self-driving car interprets the world around it. Computer vision, a subfield of artificial intelligence, plays a significant role in this process. Advanced algorithms are used to identify and classify objects, such as pedestrians, cyclists, vehicles, and road signs, in the vehicle’s field of view.
Deep learning techniques, including convolutional neural networks (CNNs), are commonly employed for object detection and recognition. These algorithms can learn from large datasets and improve their accuracy over time, enabling the car to make more informed decisions.
Decision-Making and Control
Once a self-driving car has perceived its environment, it must make decisions about how to navigate safely and efficiently. Decision-making is a complex process that involves planning routes. Predicting the behaviour of other road users, and responding to unexpected events.
Artificial intelligence plays a crucial role in decision-making. Reinforcement learning, a subset of machine learning, is used to train the car’s control system to make the best choices in various situations. The system receives rewards for good decisions and learns from its mistakes, gradually improving its decision-making capabilities.
Control algorithms are responsible for executing the vehicle’s actions, such as steering, accelerating, and braking. These algorithms use the information from the perception system to follow a planned trajectory while ensuring safety and comfort for passengers.
Safety and Redundancy
Safety is paramount in self-driving cars, and the technology is designed with redundancy and fail-safe mechanisms in mind. Autonomous vehicles are equipped with backup systems to ensure that critical functions continue to work even if a sensor or component fails.
Additionally, self-driving cars are programmed to follow strict safety protocols and ethical guidelines. For example, they prioritize the safety of passengers and pedestrians, follow traffic rules, and make ethical decisions when faced with challenging situations.
Challenges and Future Developments
Despite the rapid advancements in self-driving car technology, there are still several challenges to overcome. Some of these challenges include:
Regulatory and legal issues: Developing a comprehensive legal framework for autonomous vehicles is crucial to ensure their safe deployment.
Cybersecurity: Protecting self-driving cars from cyberattacks is a growing concern, as the vehicles rely heavily on software and communication systems.
Ethical dilemmas: Self-driving cars may face situations where ethical choices must be made, such as deciding how to prioritize the safety of passengers and pedestrians.
Conclusion
Self-driving cars represent a significant technological achievement that has the potential to transform the way we travel. The tech behind self-driving cars is a complex and interconnected system of sensors, data fusion, perception, decision-making, and safety mechanisms. As the technology continues to advance and overcome challenges, self-driving cars may soon become a common sight on our roads, Offering safer and more efficient transportation options for people around the world.
Recommended Posts
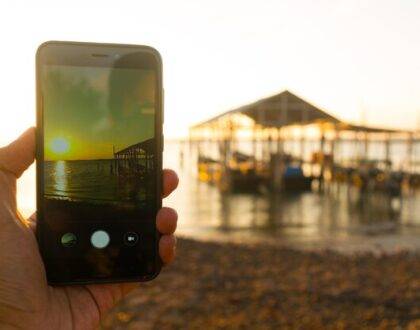
Leveraging Social Media for Wasaga Beach Tourism
December 4, 2024