The Ethical Dilemmas of AI and Machine Learning
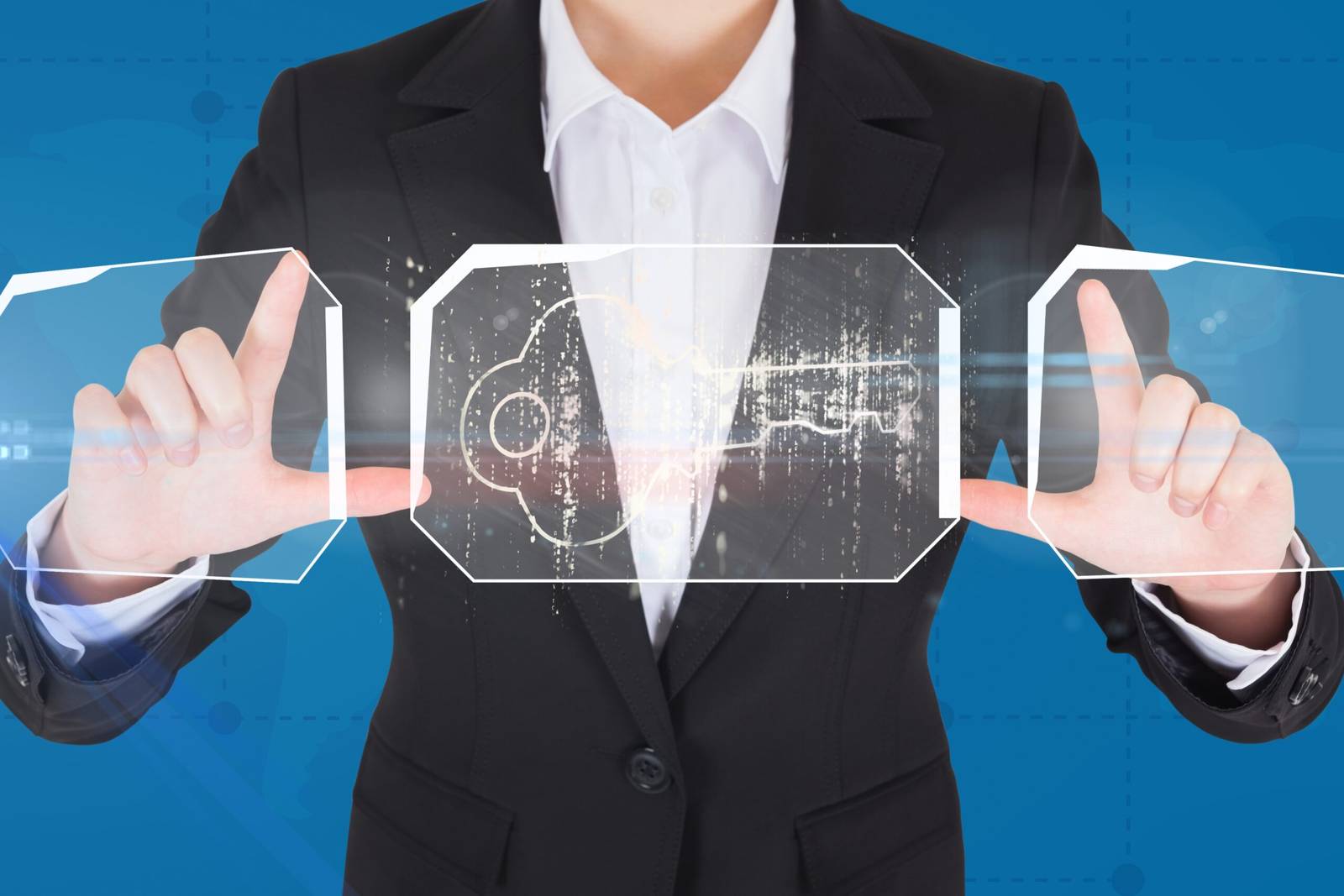

by Web Digital
Artificial Intelligence (AI) and Machine Learning (ML) have become integral parts of our daily lives, revolutionizing industries, automating tasks, and providing us with unprecedented convenience. From virtual assistants to recommendation algorithms, AI and ML technologies are increasingly embedded in the fabric of our society. However, as these technologies advance, they bring with them a host of ethical dilemmas that demand our attention. In this article, we will delve into the complex ethical challenges posed by AI and ML, exploring their impact on privacy, bias, job displacement, and accountability.
1. Privacy and Data Security
AI and ML systems often require access to vast amounts of data to function effectively. This data can include personal information, such as user behaviour, location, and even health records.
Data Breaches: The more data is collected and stored, the greater the risk of data breaches and cyberattacks. Unauthorized access to sensitive information can lead to identity theft, financial loss, and compromised privacy.
Surveillance and Privacy Invasion: AI-powered surveillance systems, like facial recognition technology, can infringe on individuals’ privacy. These systems raise concerns about mass surveillance, tracking, and the potential for abuse.
Data Exploitation: Companies may exploit user data for profit, potentially without users’ knowledge or consent. This can lead to the misuse of personal information for advertising, marketing, or even manipulation.
2. Bias and Discrimination
AI and ML systems can inherit and perpetuate biases present in the data used to train them. These biases can manifest in various forms:
Algorithmic Bias: AI algorithms may inadvertently discriminate against certain groups, such as women or minority populations. For example, biased hiring algorithms may favour male candidates over female candidates.
Reinforcement of Stereotypes: Algorithms can reinforce harmful stereotypes by reflecting societal biases present in training data. This can perpetuate discrimination and inequality.
Discriminatory Decision-Making: AI-powered decision-making systems, such as those used in criminal justice or loan approvals, can result in biased and unfair outcomes.
3. Job Displacement Machine Learning
The automation capabilities of AI and ML technologies have the potential to displace human workers in various industries. This raises concerns about unemployment, income inequality, and the impact on the workforce.
Job Loss: Automation can lead to job loss in sectors that rely heavily on repetitive and routine tasks, such as manufacturing, customer service, and data entry.
Reskilling and Upskilling: Preparing the workforce for the age of automation becomes essential. Those who are unable to reskill or upskill may be left behind, exacerbating income inequality.
4. Accountability and Decision-Making
AI and ML systems can make decisions autonomously, often without direct human intervention. This raises questions about who is accountable for the outcomes of these decisions.
Lack of Transparency: Many AI algorithms operate as “black boxes,” making it challenging to understand how they arrive at their decisions. This lack of transparency can hinder accountability.
Responsibility: Determining responsibility for AI-related decisions can be complex. Is it the developers, the users, or the AI system itself that bears responsibility for its actions?
Ethical Decision-Making: Establishing ethical guidelines for AI and ML systems is critical. Decisions made by autonomous systems should align with ethical principles and human values.
5. Ethical AI Development
The development of AI and ML systems involves making choices at various stages, from data collection and algorithm design to deployment. These choices have ethical implications:
Ethical Data Collection: Ensuring that data is collected in an ethical and responsible manner, with respect for privacy and consent, is a fundamental consideration.
Algorithm Design: Building fairness and bias mitigation into algorithms is crucial to minimize the potential for discrimination.
Human-centred AI: AI should be designed to serve human interests and values, enhancing human capabilities and decision-making rather than replacing them.
The Way Forward: Addressing Ethical Dilemmas
Addressing the ethical dilemmas posed by AI and ML requires a multifaceted approach:
Regulation and Standards: Governments and regulatory bodies must establish clear guidelines and standards for the responsible development and use of AI technologies.
Transparency and Accountability: Developers and organizations must prioritize transparency in AI systems and be accountable for their actions and outcomes.
Diversity and Inclusion: Encouraging diversity in the tech industry can help reduce biases and promote more inclusive AI development.
Education and Awareness: Raising awareness and providing education on AI ethics is vital both for developers and the general public.
Ethical AI Development: Developers should prioritize ethical considerations in every stage of AI development, from data collection to deployment.
Public Engagement: Involving the public in discussions about AI and its ethical implications can lead to more responsible AI applications.
Conclusion: Balancing Progress and Ethics
AI and Machine Learning have the potential to bring about incredible advancements and efficiencies. However, these technologies must be developed and used responsibly, with ethical considerations at the forefront. Balancing technological progress with ethical principles is a critical challenge that will shape the future of AI and its impact on society. By addressing the ethical dilemmas posed by AI and ML, we can harness the potential of these technologies while minimizing their negative consequences and ensuring a more just and equitable future.
Recommended Posts
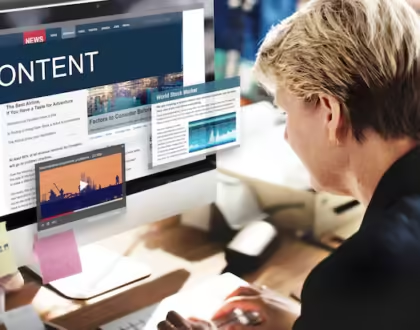
Content Marketing That Connects with Your Wallaceburg Audience
December 2, 2024